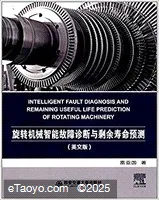
编辑推荐
《旋转机械智能故障诊断与剩余寿命预测(英文版)》通过结合机械、电子、计算机、信号处理与人工智能等学科的成果,旋转机械智能故障诊断与剩余寿命预测已然成为机械故障诊断中的重要研究方向,是大数据背景下机械故障诊断的热点内容。《旋转机械智能故障诊断与剩余寿命预测(英文版)》以转子、滚动轴承与齿轮等旋转机械为研究对象,按照“概念—理论—方法—实例”的逻辑主线,系统地介绍了旋转机械智能故障诊断与剩余寿命预测中的理论基础与前沿成果。
作者简介
Yaguo Lei is a Full Professor in the School of Mechanical Engineering at Xi'an Jiaotong University (XJTU), China, which he joined as an associate professor in 2010. Prior to that, he worked at the University of Alberta, Canada, as a postdoctoral research fellow. He also worked at the University of Duisburg-Essen,Germany, as an Mexander von Humboldt fellow in 2012. He was promoted to Full Professor in 20]3. He received the BS degree and the PhD degree both in Mechanical Engineering from XJTU, in 2002 and 2007, respectively. He is a member of the editorial boards of nine journals, including Neural Computing & Applications and Advances in MechanicalEngineering. He is also a member of ASME and a member of IEEE. He has pioneered many signal processing techniques, intelligent diagnosis methods, and remaining useful life (RUL) prediction models for rotating machinery components,such as gearboxes, bearings, and rotor systems. He is the invited author of chapters on intelligent fault diagnosis. He has published more than 80 peer-reviewed papers on signal processing, fault diagnosis and RUL prediction.
目录
Preface
Chapter 1 Introduction and Background
1.1 Introduction
1.2 Overview of PHM
1.3 Preface to Book Chapters
References
Chapter 2 Signal Processing and Feature Extraction
2.1 Introduction
2.2 Signal Preprocessing
2.3 Signal Processing in the Time Domain
2.4 Signal Processing in the Frequency Domain
2.5 Signal Processing in the Time—Frequency Domain
2.6 Conclusions
References
Chapter 3 Individual Intelligent Method—Based Fault Diagnosis
3.1 Introduction to Intelligent Diagnosis Methods
3.2 Artificial Neural Networks
3.3 Statistical Learning Theory
3.4 Deep Learning
3.5 Conclusions
References
Chapter 4 Clustering Algorithm—Based Fault Diagnosis
4.1 Introduction to Clustering Algorithm
4.2 Weighted K Nearest Neighbor—Based Fault Diagnosis
4.3 Weighted Fuzzy c—Means—Based Fault Diagnosis
4.4 Hybrid Clustering Algorithm—Based Fault Diagnosis
4.5 Conclusions
References
Chapter 5 Hybrid Intelligent Fault Diagnosis Methods
5.1 Introduction
5.2 Multipie WKNN Combination—Based Fault Diagnosis
5.3 Multiple ANFIS Hybrid Intelligent Fault Diagnosis
5.4 A Multidimensional Hybrid Intelligent Method
5.5 Conclusions
References
Chapter 6 Remaining Useful Life Prediction
6.1 Background
6.2 Data—driven Prediction Methods
6.3 Model—Based Prediction Methods
6.4 Conclusions
References
Glossary
Index
文摘
版权页:
插图:
In order to increase the reliability later the combination of multiple classifiers has been increasingly studied ro overcome the limitations of individual classifier and achieve higher classification accuracy (Samanta, 2004; Rasheed et al., 2008; Bi et al., 2008;Huenupan et al., 2008; Kittler et al., 1998; Fumera and Roli, 2005;Zio et al., 2008).It is suggested that these classifiers differing in input feature set or classification algorithm usually display complementary classification behavior.Therefore, if the classification results of multiple classifiers can be combined by integration techniques to generate the final classification result, the final result may be superior to the best result of each individual classifier on one feature set (Lei et al., 2010, 2008a, 2009, 2007).Obviously,the combination of multiple classifiers can achieve more reliable diagnosis results than single classifier.
Based on this motivation of multiple classifier combination,there exist two important factors: multiple classifiers and input feature sets.It is well known that the classifier and feature set are two key portions during intelligent fault diagnosis.Therefore, a problem arises spontaneously whether different classifiers or input feature sets will produce complementary classification performance.In order to explore this problem, this chapter mainly studies multiple classifier combination from two different aspects:classifiers and input feature sets.
《旋转机械智能故障诊断与剩余寿命预测(英文版)》通过结合机械、电子、计算机、信号处理与人工智能等学科的成果,旋转机械智能故障诊断与剩余寿命预测已然成为机械故障诊断中的重要研究方向,是大数据背景下机械故障诊断的热点内容。《旋转机械智能故障诊断与剩余寿命预测(英文版)》以转子、滚动轴承与齿轮等旋转机械为研究对象,按照“概念—理论—方法—实例”的逻辑主线,系统地介绍了旋转机械智能故障诊断与剩余寿命预测中的理论基础与前沿成果。
作者简介
Yaguo Lei is a Full Professor in the School of Mechanical Engineering at Xi'an Jiaotong University (XJTU), China, which he joined as an associate professor in 2010. Prior to that, he worked at the University of Alberta, Canada, as a postdoctoral research fellow. He also worked at the University of Duisburg-Essen,Germany, as an Mexander von Humboldt fellow in 2012. He was promoted to Full Professor in 20]3. He received the BS degree and the PhD degree both in Mechanical Engineering from XJTU, in 2002 and 2007, respectively. He is a member of the editorial boards of nine journals, including Neural Computing & Applications and Advances in MechanicalEngineering. He is also a member of ASME and a member of IEEE. He has pioneered many signal processing techniques, intelligent diagnosis methods, and remaining useful life (RUL) prediction models for rotating machinery components,such as gearboxes, bearings, and rotor systems. He is the invited author of chapters on intelligent fault diagnosis. He has published more than 80 peer-reviewed papers on signal processing, fault diagnosis and RUL prediction.
目录
Preface
Chapter 1 Introduction and Background
1.1 Introduction
1.2 Overview of PHM
1.3 Preface to Book Chapters
References
Chapter 2 Signal Processing and Feature Extraction
2.1 Introduction
2.2 Signal Preprocessing
2.3 Signal Processing in the Time Domain
2.4 Signal Processing in the Frequency Domain
2.5 Signal Processing in the Time—Frequency Domain
2.6 Conclusions
References
Chapter 3 Individual Intelligent Method—Based Fault Diagnosis
3.1 Introduction to Intelligent Diagnosis Methods
3.2 Artificial Neural Networks
3.3 Statistical Learning Theory
3.4 Deep Learning
3.5 Conclusions
References
Chapter 4 Clustering Algorithm—Based Fault Diagnosis
4.1 Introduction to Clustering Algorithm
4.2 Weighted K Nearest Neighbor—Based Fault Diagnosis
4.3 Weighted Fuzzy c—Means—Based Fault Diagnosis
4.4 Hybrid Clustering Algorithm—Based Fault Diagnosis
4.5 Conclusions
References
Chapter 5 Hybrid Intelligent Fault Diagnosis Methods
5.1 Introduction
5.2 Multipie WKNN Combination—Based Fault Diagnosis
5.3 Multiple ANFIS Hybrid Intelligent Fault Diagnosis
5.4 A Multidimensional Hybrid Intelligent Method
5.5 Conclusions
References
Chapter 6 Remaining Useful Life Prediction
6.1 Background
6.2 Data—driven Prediction Methods
6.3 Model—Based Prediction Methods
6.4 Conclusions
References
Glossary
Index
文摘
版权页:
插图:
In order to increase the reliability later the combination of multiple classifiers has been increasingly studied ro overcome the limitations of individual classifier and achieve higher classification accuracy (Samanta, 2004; Rasheed et al., 2008; Bi et al., 2008;Huenupan et al., 2008; Kittler et al., 1998; Fumera and Roli, 2005;Zio et al., 2008).It is suggested that these classifiers differing in input feature set or classification algorithm usually display complementary classification behavior.Therefore, if the classification results of multiple classifiers can be combined by integration techniques to generate the final classification result, the final result may be superior to the best result of each individual classifier on one feature set (Lei et al., 2010, 2008a, 2009, 2007).Obviously,the combination of multiple classifiers can achieve more reliable diagnosis results than single classifier.
Based on this motivation of multiple classifier combination,there exist two important factors: multiple classifiers and input feature sets.It is well known that the classifier and feature set are two key portions during intelligent fault diagnosis.Therefore, a problem arises spontaneously whether different classifiers or input feature sets will produce complementary classification performance.In order to explore this problem, this chapter mainly studies multiple classifier combination from two different aspects:classifiers and input feature sets.
ISBN | 756059X,9787560592 |
---|---|
出版社 | 西安交通大学出版社 |
作者 | 雷亚国 |
尺寸 | 16 |